Researchers explore use of machine learning to predict bulk metallic glass formation
Researchers from the Yale School of Engineering and Applied Science are analyzing the effectiveness of a machine learning tool in predicting bulk metallic glass formability.
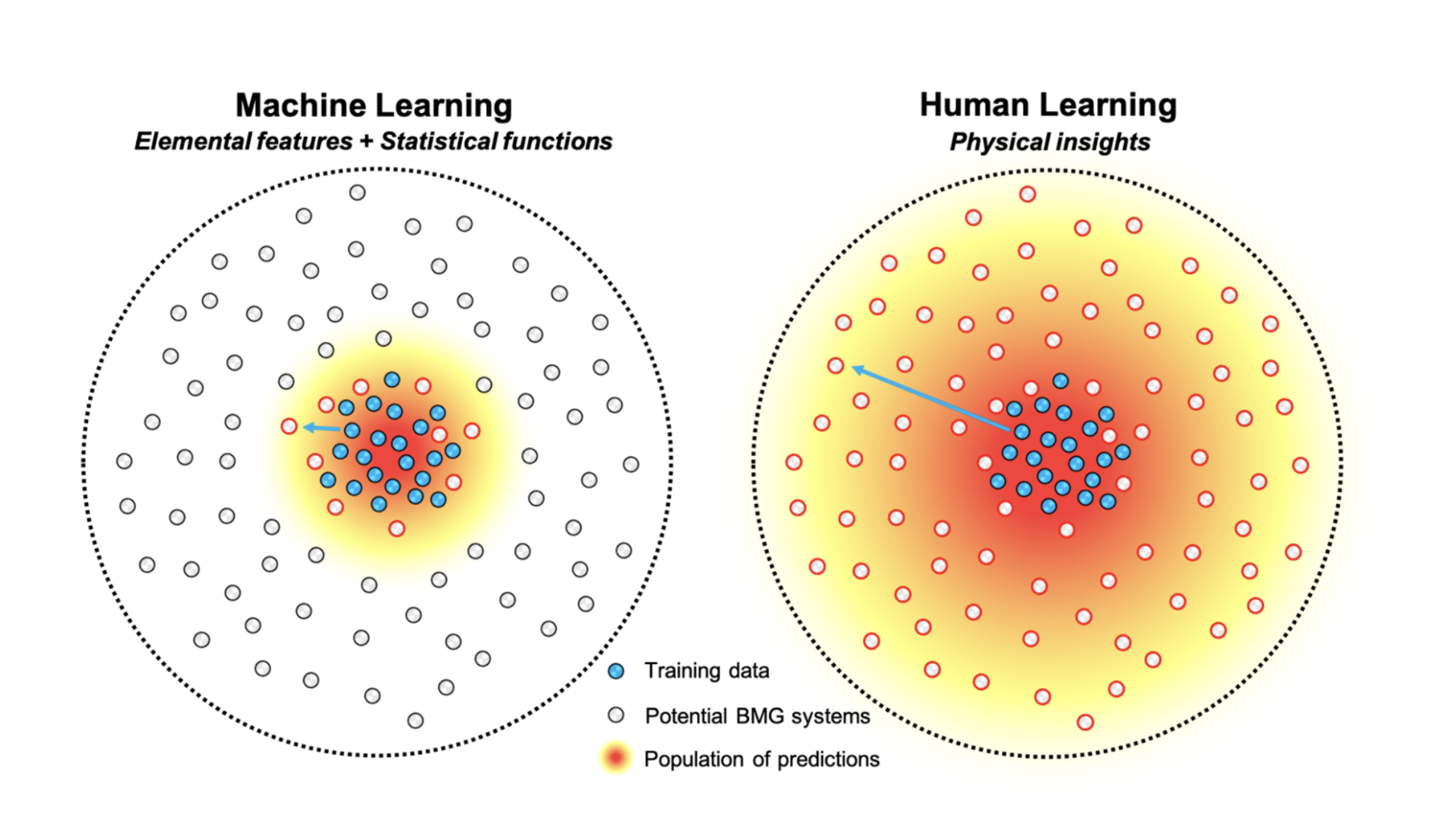
Courtesy of Guannan Liu
Machine learning has been used for a wide range of tasks such as speech recognition, fraud detection, product recommendations, image recognition and personalized medicine — nevertheless, its implementation has been limited when it comes to solving complex material science problems.
One such problem is predicting the glass-forming ability of an alloy, which is a mixture of two or more metals or of metallic and nonmetallic elements. A Yale-led study has taken this hurdle on, exploring the use of a machine learning model for the prediction of bulk metallic glass formation.
Bulk metallic glasses display unique properties including high strength, high hardness, corrosion resistance and large elastic strain limit. To predict the formability of these kinds of glasses, Yale researchers have developed a machine learning model based on 201 alloy features constructed from the combination of 31 elemental features, including atomic number, atomic weight, melting temperature, covalent radius, heat of fusion and electronegativity. This prediction was then compared with a model based on nonphysical features, as well as with a human insights-based machine learning model that they also developed.
“The nature of these different inputs is what distinguishes this work, which broadly ranges between elemental data, nonphysical data and human learned data,” said Guannan Liu GRD ’24, a doctoral student in mechanical engineering and materials science at Yale and the first author of the study.
Corey O’Hern, professor of mechanical engineering and materials science at Yale, emphasized that despite the success of machine learning tools in other fields, these approaches have so far not been able to predict a new bulk metallic glass-forming alloy. Thus, there is the opportunity for exploration ahead.
“This work begins to address that question so that new machine learning methods can be developed for bulk metallic glass design” O’Hern said.
The authors found that regardless of the nature of the data — elemental, nonphysical and human-learned — the prediction accuracy of new alloys with a similar composition from the training data-set was comparable between the models.
However, the machine learning model based on the 201 alloy features was found to yield worse results than the human-learning based model in predicting new alloys whose compositions were quite different from the training data-set.
“It reveals a very powerful idea: complex material science problems like bulk metallic glass formation require physical insights to develop effective and predictable machine learning models,” Liu said.
Since a large amount of work has focused on comparing different machine learning tools in the past, the team’s approach permitted them to compare a machine learning approach with traditional computer-aided human learning, providing a valuable insight into the applications of machine learning in material design.
Sung Woo Sohn, associate research scientist in the Department of Mechanical Engineering and Materials Science at Yale, reflected upon the difference in results between the study’s model and the human learning-based model, noting how the human learning-based model exhibited a greater ability to extrapolate than the general machine learning model, “which makes only accurate predictions in close vicinity of the known data.”
“It is important to discover the domain where machine learning is useful and equally important where it is not useful,” said Marc D. Shattuck, professor of physics at the City College of New York and a co-author of this study. “We made the first steps to define that useful domain in the area of material design.”
According to Liu, the team aims to extend the use of machine learning to other domains, such as further exploring the realm of glass formation as well as the possibilities of new bulk metallic glasses.
The study was featured in the journal Acta Materialia.