Yale researchers find certain immune cell types are predictive of COVID-19 mortality
Yale researchers applied Multiscale PHATE, a tool which was developed at Yale, to immune cell samples and found that the abundance of certain cell types can predict COVID-19 patient mortality with 83 percent accuracy.
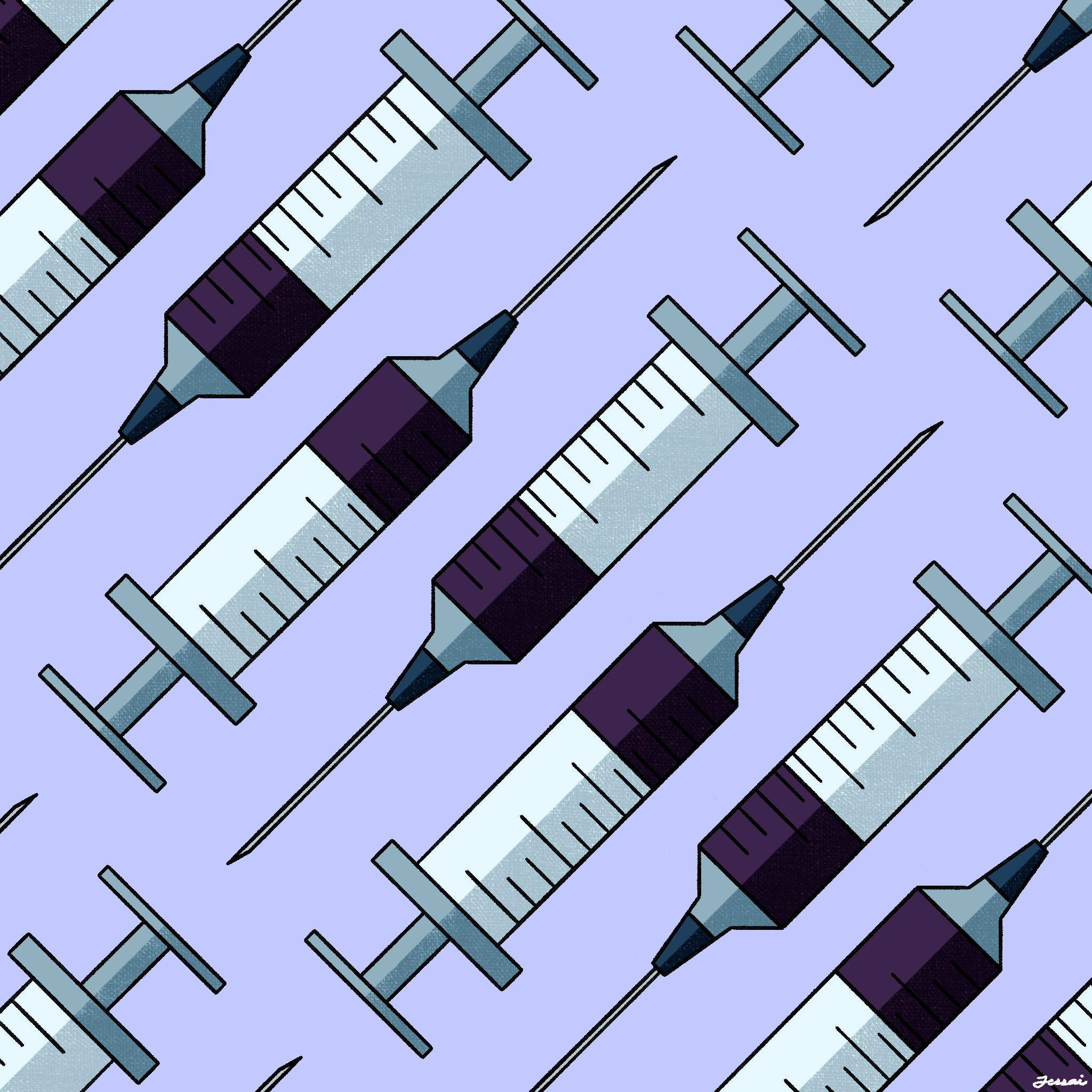
Jessai Flores
Certain immune cell types are more abundant in people who die from COVID-19, Yale researchers have discovered.
The conclusion comes after the researchers applied Multiscale PHATE, a machine learning tool, to extensive data from COVID-19 patients. Smita Krishnaswamy, professor of genetics and computer science at the Yale School of Medicine, was approached by Akiko Iwasaki, professor of immunology at the Yale School of Medicine, with a sample of immune cells from COVID-19 patients. Some of those patients died from COVID-19, while others survived. Iwasaki was curious as to why, and believed that Krishnaswamy’s Multiscale PHATE tool could comb through the data for any signs of consistent differences between survivors and people who died from COVID-19. It was found that certain immune cell types tended to be present in greater abundance in patients who died. These findings show that applying Multiscale PHATE to a COVID-19 patient’s immune cell sample can predict mortality with 83 percent accuracy.
“I contacted Dr. Krishnaswamy to help us make sense of the enormous amount of data we accumulated on COVID patients,” Iwasaki wrote in an email to the News. “They included information about their immune cells, soluble factors, antibodies and their disease course. Looking at this data with human eyes was daunting … When I met with Smita and her students to discuss the collaboration, I was delighted to hear her say, ‘We love large data.’”
Biomedical data is vague, broad and has many dimensions — also known as characteristics — Krishnaswamy explained. PHATE enables the organization and visualization of this data.
In this case, immune cells were analyzed using PHATE. PHATE analyzed each cell as an individual data point, and plotted that point based on specific characteristics of the cell, such as the presence of certain genes or proteins.
“The main idea that we have is that even though we are measuring dozens of dimensions, the data isn’t spread out in space,” explained Krishnaswamy, “but it actually forms a lower-dimensional shape that, when learned, can be really effective for learning.”
PHATE calculates the distance between each data point. This distance is representative of the similarity between two cells. Hypothetically, the greater the distance between the two cells, the less similar the cells are on the basis of the initial criteria used to plot the data points.
However, depending on the shape the data takes, this might not necessarily be the case, explained Krishnaswamy. Imagine the data as a spiral. The points next to one another forming the spiral shape are quite similar, but some distances may be calculated across the gaps in the spiral shape itself. Krishnaswamy characterized these distances across the gaps as “noise” in the data. These distances may be incorporated into the prediction of cell similarity when the points themselves represent different cell types.
To remove this noise in the data, Krishnaswamy explained that “diffusion probabilities” between each point are calculated. These probabilities are the likelihood of walking from one point to another. After the diffusion probabilities are calculated, the divergence between these probabilities is taken. Essentially, the divergence is a comparison of the probability distributions that form an interpretable data shape. This data shape is converted from 3D to 2D using a 3D scaling technique.
After applying PHATE to the data, the data goes through a process called diffusion condensation. Diffusion condensation involves condensing clusters of data points into fewer individual data points that are representative of that cluster’s properties. This creates a more understandable image from which more information can be concluded. Multiscale PHATE, a technology that was developed at Yale, is the addition of diffusion condensation to PHATE.
“Even if you have cluster structure data, you start to see substructures within it,” Krishnaswamy said. “And at some point, we really wanted to zoom in on these substructures, and that is really what gave rise to multiscale PHATE. Multiscale PHATE is really a way of taking data like [subgroups of thousands of data points] and summarizing it into clusters, giving it that ability to zoom in … when you’re zooming in, what you’re doing is going back to an earlier iteration … to see additional structure.”
Each large cluster of cells, or data points, generated from Multiscale PHATE is assigned to a cell type. Smaller clusters that are visible when zooming in are assigned to subtypes, explained Manik Kuchroo MED ’22, a doctoral candidate at the Yale School of Medicine and co-lead author on the study.
Each cluster features specific characteristics that differentiate it from the other immune cell types and subtypes plotted in the data. These differentiating characteristics were used to plot the points in the first place.
“Multiscale PHATE addresses what cell types, or sub-cell types, would be important to look at to get a picture of what cells are leading to death in patients,” said Kuchroo.
This process of plotting immune cells as data points and determining which cell types are most abundant was repeated for many COVID-19 patients. Kuchroo explained that, in patients who die from COVID-19, they found a high abundance of granulocytes and monocytes.
Therefore, granulocytes and monocytes are immune cells that have a strong association with COVID-19 mortality. On the contrary, the presence of T-cells seemed to have little correlation with COVID-19 mortality.
“[Kuchroo] found that neutrophils, which are [the] clean-up crew that removes dead cells, had the highest mortality score, meaning that they were most associated with lethal COVID,” Iwasaki wrote. “This made sense because neutrophils are known to spew out toxic factors during viral infection that [are] harmful to the host. On the other hand, T-cells capable of killing virally infected cells were the least associated with mortality. Among the T-cell subsets, however, there were some bad players. One called Th17* had the worst mortality score of all T-cell subsets, suggesting their pathological involvement.”
In speaking on the implications of these findings, Krishnaswamy explained that running Multiscale PHATE on a patient’s immune cells can help determine the best path of treatment. Furthermore, this technology has the potential to be applied to many other diseases.
According to Iwasaki, these findings show that lethal COVID-19 infection is likely not caused by the virus itself, but may be influenced by the host’s malfunctioning immune response. She suggested that treatment targeting these “rogue” immune system factors may be useful in preventing fatalities.
There have been a total of 963,244 deaths due to COVID-19 in the United States as of March 15, 2022.